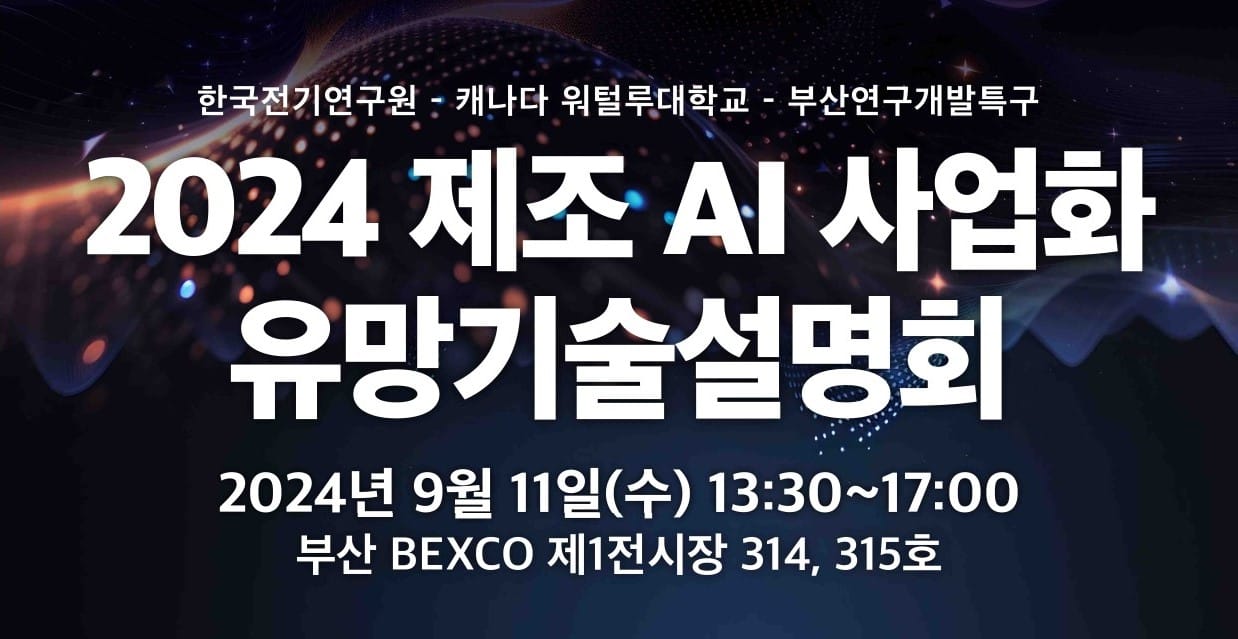
Session 3️⃣
Simulation for Predicting Machined Product Quality
세션 3. AI 기반 시뮬레이션을 이용한 가공품 품질 예측 기술
워털루대학교 한정우 박사
Wouldn't it be great if we could judge the quality of machined products just by looking at them? Unfortunately, reality isn't that simple. Traditionally, Non-Destructive Testing (NDT) methods have been used to detect defects in materials and parts. However, as the need for precise analysis increases, the computational load and costs also rise significantly.
A method used to inspect the internal structure and identify defects in materials or products without causing any damage to them.
재료나 제품을 손상시키지 않고 공업제품 내부의 가공이나 결함 등을 검사하는 비파괴 검사 방법 (초음바, 방사선, 와전류 등) 입니다.
To address this challenge, Dr. Jungwoo Han from the University of Waterloo introduced a quality prediction model based on Physics-Informed Neural Networks (PINN). This innovative approach aims to reduce the time and costs associated with NDT by leveraging advanced AI techniques to predict the quality of machined products more efficiently.
Incorporates various physical constraints into the training process of deep learning models to solve complex problems effectively.
다양한 물리적인 제한조건을 Deep Learning 모델 학습과정에 포함하여 해결하는 물리정보 신경망
만들어진 가공품을 보기만 해도 그 완성도를 판단할 수 있다면 얼마나 좋을까요?
그러나 현실은 그렇지 않습니다. 그래서 기존에는 NDT 기술을 활용하여 재료나 부품의 결함을 확인하곤 했습니다.
그러나 NDT 방식은 정확하게 분석하려 할수록 계산의 양이 급격하게 많아진다는 문제가 있어 이를 해결하여 시간과 비용을 절약하기 위해 워털루대학교의 한정우 박사님은 PINN 기술을 기반으로 한 품질 예측 모델을 소개하였습니다.
Practical Application Cases
실제 적용 사례
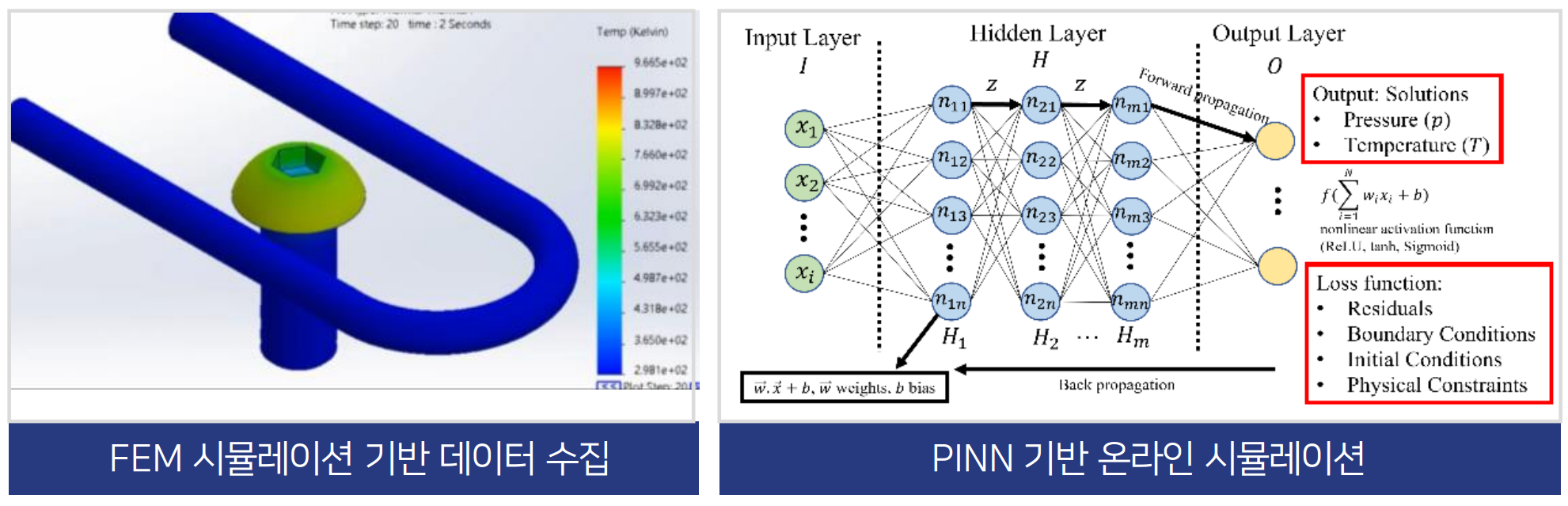
High-Frequency Heat Treatment System: Specialized Heat Treatment Provider
Heat treatment processes are crucial for altering material properties, such as enhancing durability. However, factors like temperature and time can significantly impact the final product quality. Therefore, setting the initial conditions for the process and accurately predicting the quality based on those conditions is essential.
This quality prediction technology utilizes ultrasound signals and Finite Element Analysis (FEA) to gather data grounded in actual physical laws. By employing a PINN-based simulation model, it can predict temperature and hardness, ensuring more reliable and consistent outcomes in heat treatment processes.
고주파 열처리 시스템 : 부품 열처리 전문업체
열처리 공정은 내구성을 높이는 등 재료의 특성을 바꾸는 데에 매우 중요한 역할을 하지만, 온도, 시간 등 다양한 요소에 의해 결과물의 품질이 저하될 수 있습니다. 따라서 공정에 대한 초기 조건을 설정하고 그 조건을 기반으로 품질을 정확하게 예측하는 것이 중요합니다.
이 가공품 품질 예측 기술은 초음파 신호를 유한 요소 해석(FEA)을 통해 실제 물리법칙을 기반으로 한 데이터를 획득하고, PINN 기반의 시뮬레이션 모델을 활용하여 온도와 경도를 예측할 수 있습니다.
Written by Jaemin Kim
DX & AI Researcher
ORSKOREA
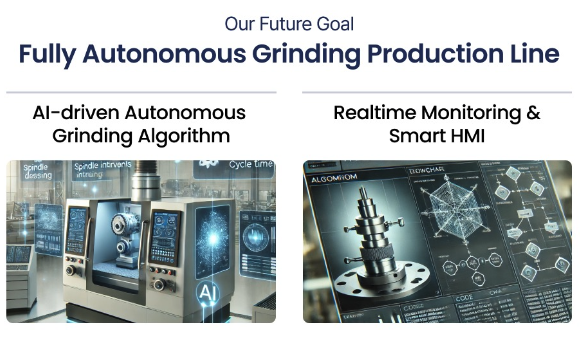
As ORSKOREA continues to innovate in AI-driven manufacturing, our ultimate vision is to develop fully autonomous grinding machines that operate with unparalleled precision and efficiency. Beyond that, we are striving to design advanced robots capable of independently managing and enhancing production processes in the near future.
This report is just one step in our journey toward a smarter, more connected manufacturing industry. Stay tuned for our next session, where we’ll dive deeper into how AI is shaping the future of machining and automation.
오알에스코리아는 AI 기반 제조 혁신을 지속하며, 완전 자율 제어 연삭기를 개발하는 것을 궁극적인 목표로 삼고 있습니다. 더 나아가, 미래에는 생산 공정을 독립적으로 관리하고 개선할 수 있는 첨단 로봇 설계에도 도전하고자 합니다.
이번 보고서는 더 스마트하고 연결된 제조 산업을 향한 여정의 한 걸음에 불과합니다. 다음 세션에서는 AI가 가공 및 자동화의 미래를 어떻게 만들어가는지에 대해 더 깊이 다룰 예정이니 기대해 주세요.